(2019-Feb-18) With Azure Data Factory (ADF) continuous integration, you help your team to collaborate and develop data transformation solutions within the same data factory workspace and maintain your combined development efforts in a central code repository. Continuous delivery helps to build and deploy your ADF solution for testing and release purposes. Basically, the CI/CD process helps to establish a good software development practice and aims to build a healthy relationship between development, quality assurance, and other supporting teams.
Update 2020-Mar-15: Part 2 of this blog post published in 2020-Jan-28:
http://datanrg.blogspot.com/2020/01/continuous-integration-and-delivery.html
Update 2019-Jun-24:
Video recording of my webinar session on Continuous Integration and Delivery (CI/CD) in Azure Data Factory at a recent PASS Cloud Virtual Group meeting.
Back in my SQL Server Integration Services (SSIS) heavy development times, SSIS solution deployment was a combination of building ispac files and running a set of PowerShell scripts to deploy this file to SSIS servers and update required environmental variables if necessary.
Microsoft introduced DevOps culture for software continuous integration and delivery - https://docs.microsoft.com/en-us/azure/devops/learn/what-is-devops, and I really like their starting line, "DevOps is the union of people, process, and products to enable continuous delivery of value to our end users"!
Recently, they've added a DevOps support for Azure Data Factory as well - https://docs.microsoft.com/en-us/azure/data-factory/continuous-integration-deployment and those very steps I tried to replicate in this blog post.
My ADF pipeline use-case to deploy
So, here is my use case: I have a pipeline in my Azure Data Factory synced in GitHub (my Development workspace) and I want to be able to Test it in a separate environment and then deploy to a Production environment.
In my ADF I have a template pipeline solution "Copy multiple files containers between File Stores" that I used to copy file/folders from one blob container to another one.
In my Azure development resource group, I created three artifacts (which may vary depending on your own development needs):
- Key Vault, to store secret values
- Storage Account, to keep my testing files in blob containers
- Data Factory, the very thing I tend to test and deploy
Creating continuous integration and delivery (CI/CD) pipeline for my ADF
Step 1: Integrating your ADF pipeline code to source control (GitHub)
In my previous blog post (Azure Data Factory integration with GitHub) I had already shown a way to sync your code to the GitHub. For the continuous integration (CI) of your ADF pipeline, you will need to make sure that along with your development (or feature) branch you will need to publish your master branch from your Azure Data Factory UI. This will create an additional adf_publish branch of your code repository in GitHub.
As Gaurav Malhotra (Senior Product Manager at Microsoft) has commented about this code branch, "The adf_publish branch is automatically created by ADF when you publish from your collaboration branch (usually master) which is different from adf_publish branch... The moment a check-in happens in this branch after you publish from your factory, you can trigger the entire VSTS release to do CI/CD"
Publishing my ADF code from master to adf_publish branch automatically created two files:
Template file (ARMTemplateForFactory.json): Templates containing all the data factory metadata (pipelines, datasets etc.) corresponding to my data factory.
Configuration file (ARMTemplateParametersForFactory.json): Contains environment parameters that will be different for each environment (Development, Testing, Production etc.).
Step 2: Creating a deployment pipeline in Azure DevOps
2.a Access to DevOps: If you don't have a DevOps account, you can start for free - https://azure.microsoft.com/en-ca/services/devops/ or ask your DevOps team to provide you with access to your organization build/release environment.
2.b Create new DevOps project: by clicking [+ Create project] button you start creating your build/release solution.
2.c Create a new release pipeline
In a normal software development cycle, I would create a build pipeline in my Azure DevOps project, then archive the built files and use those deployment files for further release operations. As Microsoft team indicated in their CI/CD documentation page for Azure Data Factory, the Release pipeline is based on the source control repository, thus the Build pipeline is omitted; however, it's a matter of choice and you can always change it in your solution.
Select [Pipelines] > [Release] and then click the [New pipeline] button in order to create a new release pipeline for your ADF solution.
2.d Create Variable groups for your solution
This step is optional, as you can add variables directly to your Release pipelines and individual releases. But for the sake of reusing some of the generic values, I created three groups in my DevOps project with the same (Environment) variable for each of these groups with ("dev", "tst", "prd") corresponding values within.
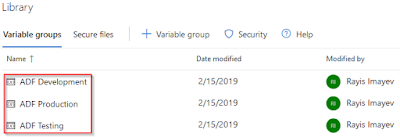
2.e Add an artifact to your release pipeline
In the Artifact section, I set the following attributes:
- Service Connection my GitHub account
- GitHub repository for my Azure Data Factory
- Default branch (adf_branch) as a source for my release processing
- Alias (Development) as it is the beginning of everything
2.f Add new Stage to your Release pipeline
I click [+ Add a stage] button, select "Empty Job" template and name it as "Testing". Then I add two agent jobs from the list of tasks:
- Azure Key Vault, to read Azure secret values and pass them for my ADF ARM template parameters
- Azure Resource Group Deployment, to deploy my ADF ARM template
In my real job ADF projects, DevOps Build/Release pipelines are more sophisticated and managed by our DevOps and Azure Admin teams. Those pipelines may contain multiple PowerShell and other Azure deployment steps. However, for my blog post purpose, I'm only concerned to deploy my data factory to other environments.
For the (Azure Key Vault) task, I set "Key vault" name to this value: azu-caes-$(Environment)-keyvault, which will support all three environments' Key Vaults depending on the value of the $(Environment) group variable {dev, tst, prd}.
For the (Azure Resource Group Deployment) task, I set the following attributes:
- Action: Create or update resource group
- Resource group: azu-caes-$(Environment)-rg
- Template location: Linked artifact
- Template: in my case the linked ART template file location is
$(System.DefaultWorkingDirectory)/Development/azu-eus2-dev-datafactory/ARMTemplateForFactory.json
- Template parameters: in my case the linked ARM template parameters file location is
$(System.DefaultWorkingDirectory)/Development/azu-eus2-dev-datafactory/ARMTemplateParametersForFactory.json
- Override template parameters: some of my ADF pipeline parameters I either leave blank or with default value, however, I specifically set to override the following parameters:
+ factoryName: $(factoryName)
+ blob_storage_ls_connectionString: $(blob-storage-ls-connectionString)
+ AzureKeyVault_ls_properties_typeProperties_baseUrl: $(AzureKeyVault_ls_properties_typeProperties_baseUrl)
- Deployment mode: Incremental
2.g Add Release Pipeline variables:
To support reference of variables in my ARM template deployment task, I add the following variables within the "Release" scope:
- factoryName
- AzureKeyVault_ls_properties_typeProperties_baseUrl
2.h Clone Testing stage to a new Production stage:
2.i Link Variable groups to Release stages:
A final step in creating my Azure Data Factory release pipeline is that I need to link my Variable groups to corresponding release stages. I go to the Variables section in my Release pipeline and select Variable groups, by clicking [Link variable group] button I choose to link a variable group to a stage so that both of my Testing and Production sections would look the following way:
After saving my ADF Release pipeline, I keep my fingers crossed :-) It's time to test it!
Step 3: Create and test a new release
I click [+ Create a release] button and start monitoring my ADF release process.
After it had been successfully finished, I went to check my Production Data Factory (azu-eus2-prd-datafactory) and was able to find my deployed pipeline which was originated from the development environment. And I successfully test this Production ADF pipeline by executing it.
One thing to note, that all data connections to Production Key Vault and Blob Storage account have been properly deployed though by ADF release pipeline (no manual interventions).
Step 4: Test CI/CD process for my Azure Data Factory
Now it's time to test a complete cycle of committing new data factory code change and deploying it both to Testing and Production environments.
1) I add a new "Wait 5 seconds" activity task to my ADF pipeline and saving it to my development branch of the (azu-eus2-dev-datafactory) development data factory instance.
2) I create a pull request and merge this change to the master branch of my GitHub repository
3) Then I publish my new ADF code change from master to the adf_publish branch.
4) And this automatically triggers my ADF Release pipeline to deploy this code change to Testing and then Production environments.
Don't forget to enable "Continues deployment trigger" on your Artifact release pipeline and select the final 'adf-publish' code branch as your filter. Otherwise, all of your GitHub branches code commitment might trigger release/deployment process.
5) And after the new release is successfully finished, I can check that the new "Wait" activity task appears in the Production data factory pipeline:
Summary:
1) I feel a bit exhausted after writing such a long blog post. I hope you will find it helpful in your own DevOps pipelines for your Azure Data Factory solutions.
2) After seeing a complete CI/CD cycle of my Azure Data Factory where the code was changed, integrated into the main source code repository and automatically deployed, I can confidently say that my data factory has been successfully released! :-)
Link to my GitHub repository with the ADF solution from this blog post can be found here: https://github.com/NrgFly/Azure-DataFactory-CICD
And happy data adventure!
Update 2020-Mar-15: Part 2 of this blog post published in 2020-Jan-28:
http://datanrg.blogspot.com/2020/01/continuous-integration-and-delivery.html
Update 2019-Jun-24:
Video recording of my webinar session on Continuous Integration and Delivery (CI/CD) in Azure Data Factory at a recent PASS Cloud Virtual Group meeting.
Back in my SQL Server Integration Services (SSIS) heavy development times, SSIS solution deployment was a combination of building ispac files and running a set of PowerShell scripts to deploy this file to SSIS servers and update required environmental variables if necessary.
Microsoft introduced DevOps culture for software continuous integration and delivery - https://docs.microsoft.com/en-us/azure/devops/learn/what-is-devops, and I really like their starting line, "DevOps is the union of people, process, and products to enable continuous delivery of value to our end users"!
Recently, they've added a DevOps support for Azure Data Factory as well - https://docs.microsoft.com/en-us/azure/data-factory/continuous-integration-deployment and those very steps I tried to replicate in this blog post.
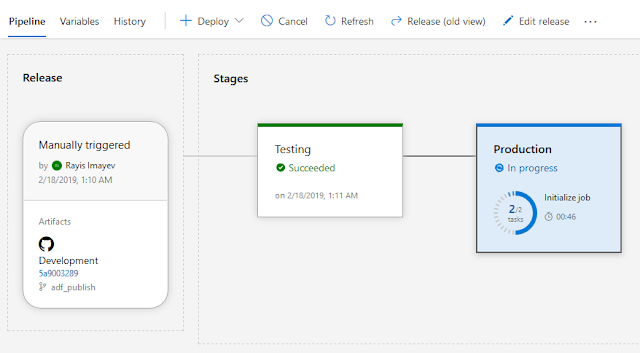
My ADF pipeline use-case to deploy
So, here is my use case: I have a pipeline in my Azure Data Factory synced in GitHub (my Development workspace) and I want to be able to Test it in a separate environment and then deploy to a Production environment.
Development > Testing > Production
In my ADF I have a template pipeline solution "Copy multiple files containers between File Stores" that I used to copy file/folders from one blob container to another one.

In my Azure development resource group, I created three artifacts (which may vary depending on your own development needs):
- Key Vault, to store secret values
- Storage Account, to keep my testing files in blob containers
- Data Factory, the very thing I tend to test and deploy

Creating continuous integration and delivery (CI/CD) pipeline for my ADF
Step 1: Integrating your ADF pipeline code to source control (GitHub)
In my previous blog post (Azure Data Factory integration with GitHub) I had already shown a way to sync your code to the GitHub. For the continuous integration (CI) of your ADF pipeline, you will need to make sure that along with your development (or feature) branch you will need to publish your master branch from your Azure Data Factory UI. This will create an additional adf_publish branch of your code repository in GitHub.
development > master > adf_publish
As Gaurav Malhotra (Senior Product Manager at Microsoft) has commented about this code branch, "The adf_publish branch is automatically created by ADF when you publish from your collaboration branch (usually master) which is different from adf_publish branch... The moment a check-in happens in this branch after you publish from your factory, you can trigger the entire VSTS release to do CI/CD"
Publishing my ADF code from master to adf_publish branch automatically created two files:
Template file (ARMTemplateForFactory.json): Templates containing all the data factory metadata (pipelines, datasets etc.) corresponding to my data factory.
Configuration file (ARMTemplateParametersForFactory.json): Contains environment parameters that will be different for each environment (Development, Testing, Production etc.).

Step 2: Creating a deployment pipeline in Azure DevOps
2.a Access to DevOps: If you don't have a DevOps account, you can start for free - https://azure.microsoft.com/en-ca/services/devops/ or ask your DevOps team to provide you with access to your organization build/release environment.
2.b Create new DevOps project: by clicking [+ Create project] button you start creating your build/release solution.
2.c Create a new release pipeline
In a normal software development cycle, I would create a build pipeline in my Azure DevOps project, then archive the built files and use those deployment files for further release operations. As Microsoft team indicated in their CI/CD documentation page for Azure Data Factory, the Release pipeline is based on the source control repository, thus the Build pipeline is omitted; however, it's a matter of choice and you can always change it in your solution.
Select [Pipelines] > [Release] and then click the [New pipeline] button in order to create a new release pipeline for your ADF solution.
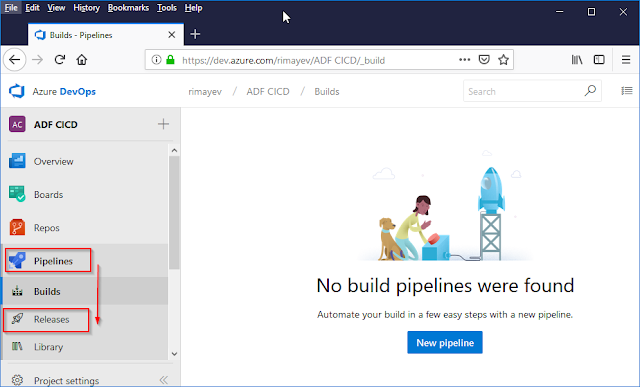
2.d Create Variable groups for your solution
This step is optional, as you can add variables directly to your Release pipelines and individual releases. But for the sake of reusing some of the generic values, I created three groups in my DevOps project with the same (Environment) variable for each of these groups with ("dev", "tst", "prd") corresponding values within.
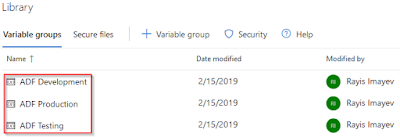
2.e Add an artifact to your release pipeline
In the Artifact section, I set the following attributes:
- Service Connection my GitHub account
- GitHub repository for my Azure Data Factory
- Default branch (adf_branch) as a source for my release processing
- Alias (Development) as it is the beginning of everything
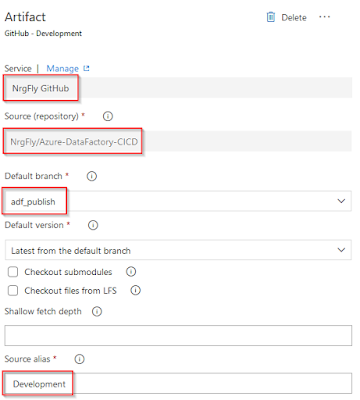
2.f Add new Stage to your Release pipeline
I click [+ Add a stage] button, select "Empty Job" template and name it as "Testing". Then I add two agent jobs from the list of tasks:
- Azure Key Vault, to read Azure secret values and pass them for my ADF ARM template parameters
- Azure Resource Group Deployment, to deploy my ADF ARM template

In my real job ADF projects, DevOps Build/Release pipelines are more sophisticated and managed by our DevOps and Azure Admin teams. Those pipelines may contain multiple PowerShell and other Azure deployment steps. However, for my blog post purpose, I'm only concerned to deploy my data factory to other environments.
For the (Azure Key Vault) task, I set "Key vault" name to this value: azu-caes-$(Environment)-keyvault, which will support all three environments' Key Vaults depending on the value of the $(Environment) group variable {dev, tst, prd}.

For the (Azure Resource Group Deployment) task, I set the following attributes:
- Action: Create or update resource group
- Resource group: azu-caes-$(Environment)-rg
- Template location: Linked artifact
- Template: in my case the linked ART template file location is
$(System.DefaultWorkingDirectory)/Development/azu-eus2-dev-datafactory/ARMTemplateForFactory.json
- Template parameters: in my case the linked ARM template parameters file location is
$(System.DefaultWorkingDirectory)/Development/azu-eus2-dev-datafactory/ARMTemplateParametersForFactory.json
- Override template parameters: some of my ADF pipeline parameters I either leave blank or with default value, however, I specifically set to override the following parameters:
+ factoryName: $(factoryName)
+ blob_storage_ls_connectionString: $(blob-storage-ls-connectionString)
+ AzureKeyVault_ls_properties_typeProperties_baseUrl: $(AzureKeyVault_ls_properties_typeProperties_baseUrl)
- Deployment mode: Incremental

2.g Add Release Pipeline variables:
To support reference of variables in my ARM template deployment task, I add the following variables within the "Release" scope:
- factoryName
- AzureKeyVault_ls_properties_typeProperties_baseUrl
2.h Clone Testing stage to a new Production stage:
By clicking the "Clone" button in the "Testing" stage I create a new release stage and name it as "Production". I will keep both tasks within this new stage unchanged, where the $(Environment) variable should do the whole trick of switching between two stages.
2.i Link Variable groups to Release stages:
A final step in creating my Azure Data Factory release pipeline is that I need to link my Variable groups to corresponding release stages. I go to the Variables section in my Release pipeline and select Variable groups, by clicking [Link variable group] button I choose to link a variable group to a stage so that both of my Testing and Production sections would look the following way:

After saving my ADF Release pipeline, I keep my fingers crossed :-) It's time to test it!
Step 3: Create and test a new release
I click [+ Create a release] button and start monitoring my ADF release process.

After it had been successfully finished, I went to check my Production Data Factory (azu-eus2-prd-datafactory) and was able to find my deployed pipeline which was originated from the development environment. And I successfully test this Production ADF pipeline by executing it.
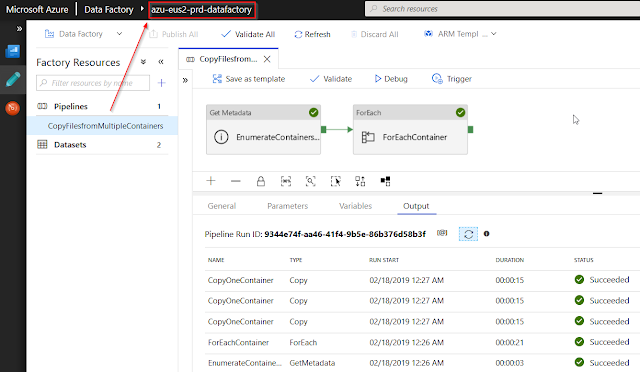
One thing to note, that all data connections to Production Key Vault and Blob Storage account have been properly deployed though by ADF release pipeline (no manual interventions).
Step 4: Test CI/CD process for my Azure Data Factory
Now it's time to test a complete cycle of committing new data factory code change and deploying it both to Testing and Production environments.
1) I add a new "Wait 5 seconds" activity task to my ADF pipeline and saving it to my development branch of the (azu-eus2-dev-datafactory) development data factory instance.
2) I create a pull request and merge this change to the master branch of my GitHub repository
3) Then I publish my new ADF code change from master to the adf_publish branch.
4) And this automatically triggers my ADF Release pipeline to deploy this code change to Testing and then Production environments.

Don't forget to enable "Continues deployment trigger" on your Artifact release pipeline and select the final 'adf-publish' code branch as your filter. Otherwise, all of your GitHub branches code commitment might trigger release/deployment process.
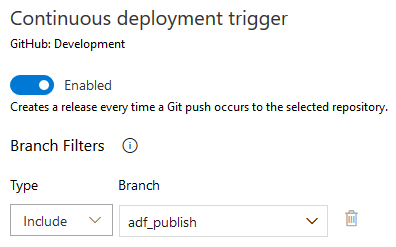
5) And after the new release is successfully finished, I can check that the new "Wait" activity task appears in the Production data factory pipeline:
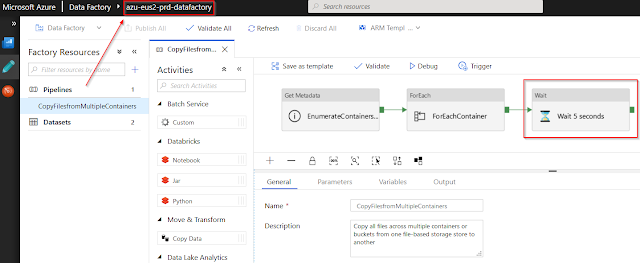
Summary:
1) I feel a bit exhausted after writing such a long blog post. I hope you will find it helpful in your own DevOps pipelines for your Azure Data Factory solutions.
2) After seeing a complete CI/CD cycle of my Azure Data Factory where the code was changed, integrated into the main source code repository and automatically deployed, I can confidently say that my data factory has been successfully released! :-)
Link to my GitHub repository with the ADF solution from this blog post can be found here: https://github.com/NrgFly/Azure-DataFactory-CICD
And happy data adventure!
You've used the environment parameter in the Resource Group. But what if there are 2 Data Factories in the same Resource Group? And only the name is different like xx-dev-xx or xx-uat-xx. You're variables are related to the Resource Group Name.
ReplyDeleteThanks for your comment. Yes, different environment Azure objects should stay in different resource groups, otherwise you don't need set $(Environment) variable, and a hard-coded resource group name would work, which I don't recommend.
DeleteI created 3 different resource groups: azu-caes-dev-rg, azu-caes-tst-rg, azu-caes-prd-rg.
And within each groups I created 3 Azure resource objects:
- Key Vault, azu-caes-$(Environment)-keyvault
- Storage Account, azucaes$(Environment)storageaccount
- Data Factory, azu-eus2-$(Environment)-datafactory
9 objects in total for my blog post use-case. Your case could be different.
@Unknown, As Rayis mentioned, using resource groups and subscriptions are common methods to delineate environments (subscriptions that is). Resource groups are for related objects that have a similar lifecycle and should be deployed/updated together.
DeleteRayis with more than one developer or several projects with many DF pipelines and DF datasets using data factory. what is the best way to separate projects allow some assets to be developed while other are moved through the a devops pipeline to release.
ReplyDeleteHi, @Ozhug, treat your Data Factory as one complex SSIS project, where each of your ADF pipelines could be considered as a set of SSIS packages. For a team development in Data Factory, each developer could create a feature branch from a Git source control, and after finishing development work for a particular branch their code changes could be merged with a development or master-release branch, which then will be a candidate for a release and deployment using DevOps.
Delete@Rayis, if there are multiple devs in a team, and each creates its own development branch, they can certainly work on the feature branch from a code perspective, editing the JSON files directly, but how can they run their development branch data factory using the Azure DF UI, if there are only 3 environments (dev, test, prod)? Do developers change only JSON files?
DeleteDeveloper don't have to change ADF code in JSON files directly, that can use ADF UI for this.
DeleteDeveloper can work in their own feature GitHub branches and then pull those changes back to the main Development branch still using the ADF UI.
Development GitHub branch is separate from the Dev,Test,Prod or other ADF live environments. You need to provision ADF resources in all those environments your self. And then you will need to create a DevOps pipeline to deploy your GitHub code to all environments. Please don't mix Development GitHub branch of your source code with the Development environment of your Data Factory, they're separate.
Developer don't have to change ADF code in JSON files directly, that can use ADF UI for this. Developer can work in their own feature GitHub branches and then pull those changes back to the main Development branch still using the ADF UI.
DeleteSaw the response above Rayis. But when we use ADF integrated with GIT for development, It will not be cherry picking like above right?.
Please don't mix Development GitHub branch of your source code with the Development environment of your Data Factory, they're separate
How to keep them separate?.
We have ADF running in our DEV and higher environments. The DEV envorionment is backed by a GIT repo and releases are automated via Azure DevOps / ARM templates.
ReplyDeleteNow, we are cleaning up some object which are not in use anymore. Several pipelines have to be deleted. But we're getting failures when we try to publish. The failure has a description like: "The document cannot be deleted since it is refernced by....". In some cases, this is not true. In fact, the so called referencing objects don't even exist anymore. The ARM files have no entry with the given name...
Does this sound familair to anybody? Does anyone know how to fix this?
Yes, it does sound familiar. My coworker (Patrick) helped me to understand this, so I will give him full credits.
DeleteSince ARM deploys in incremental mode, it does not clean up after itself for objects that have been deleted. The script deals with that:
https://docs.microsoft.com/en-us/azure/data-factory/continuous-integration-deployment#sample-script-to-stop-and-restart-triggers-and-clean-up
Patrick made improvements to that script.
You just need to add this script as PowerShell step before deploying your ARM template.
Hi Rayis, we are already using this. And this works for deleting the objects. But never the less publishing has a certain check what detects a reference what does not exists any more.
DeleteMy previous comment was not entirely correct after really fixing the issue. The issue was caused by old Pipelines in ADF what where created pre GIT. There where references to existing pipelines but these where not in de GIT environment, so wherent cleaned by GIT. After deleting them in our ADF dev environment by hand the issue was fixed. And for releasing to DAP we use the powershell script provided by microsoft with some adjustments.
DeleteWould you please elaborate on Agent job / Agent pool setting?
ReplyDeleteYes, Microsoft did a good job introducing time schedule triggers for ADF pipelines, similarly as for Agent jobs for SSIS packages).
Deletehttps://docs.microsoft.com/en-us/azure/data-factory/how-to-create-schedule-trigger
Thanks for your quick reply. But this is not what I meant. When I create a release pipeline the first thing which I need to configure is an "Agent job" and you have to select one of different options ( Default (Default), Hosted (Hosted), Hosted macOS (Hosted macOS), Hosted VS2017 (Hosted VS2017), etc.)
DeleteI want to know what is the role of this and in terms of deploying ADF what is the best option and how to configure it.
My apologies, I misunderstood your question. I will admit that before creating DevOps pipeline in Azure I knew nothing about Agent and Agent pools. During our training with Microsoft, they instructed us that for initial use build and release pipelines Hosted VS2017 is sufficient, it's a shared environment to host resources and logs related to DevOps pipelines processing. During testing with more people in our team, we discovered the Hosted VS2017 may become slow, therefore dedicated VM would help. At my company, we have a DevOps team who manages those agent pools VMs and in case of a detailed investigation, we may request them to provide us with some additional logging information after each of the release pipeline running. I hope this helps.
DeleteOtherwise, Microsoft documentation sites are very informative as well: https://docs.microsoft.com/en-us/azure/devops/pipelines/agents/pools-queues?view=azure-devops
Hello Rayis,
ReplyDeleteI'm stuck at creating the 2.g. because i dont have any KeyVault for ADF.
its nice work. if you make video of this complete process and upload that helps us little bit more clear.
could you please help on this to complete my task.
Regards,
Rakesh Dontha
rakeshdontha34@gmail.com
can you suggest me what are the variables to be created in Keyvault for ADF for Dev and test. but i didnt used keyvault in my ADF because these ADF's are old.
ReplyDeleteIf you don't have KeyVault, it won't heart to creating one. It's a good and secure place to keep connection strings and other access related items there that you can securely reference during building data connectors in your ADF pipelines.
DeleteSo for my blog post, I created two secrets:
1) blob-storage-key: to connect to blob storage from my ADF pipeline
2) blob-storage-ls-connectionString: which I use in my build pipeline and pass for the same name template parameter of my deployment ARM template. You can see them all in my adf_publish GitHub branch:
https://github.com/NrgFly/Azure-DataFactory-CICD/blob/adf_publish/azu-eus2-dev-datafactory/ARMTemplateForFactory.json
Let me know if this helps you.
Thanks Rayis,
DeleteThanks for your active response. what are keys to be created for azure sqlDB? Is one sql connectionstring is enough to execute my process?
Try connection string or password and decide which one you will use.
DeleteI have created the secret key for connection string. but im unable to connect to LinkedService in ADF connection. im getting access denied error while testing the connection string. could you please help me out.
DeleteERROR: Failed to get the secret from key vault, secretName: ****, secretVersion: , vaultBaseUrl: https://****.vault.azure.net/. The error message is: Access denied
what changes i need to do and where.
You need to grant ADF to access your Key Vault. Please read the steps in this documentation page:
Deletehttps://docs.microsoft.com/en-us/azure/data-factory/store-credentials-in-key-vault
Hello Rayis,
Deletei'm out of that error now. now running into new error.
while im releasing the build getting following MIS error.
Could not fetch access token for Managed Service Principal. Please configure Managed Service Identity (MSI) for virtual machine.
could you please help me on this
It's hard to tell without knowing what step is it failing. Did you grant your VSTS pipelines to access your Key Vault?
DeletePlease check if you followed these steps:
https://github.com/MicrosoftDocs/azure-docs/blob/master/articles/data-factory/continuous-integration-deployment.md#grant-permissions-to-the-azure-pipelines-agent
granting access from adf to the key vault is the answer indeed
ReplyDeleteThis comment has been removed by the author.
ReplyDeleteHello Rayis,
ReplyDeleteFirstly, Appreciate you for creating this wonderful post as it is more elaborate and detailed!
Now I got one question for you. what if we have a separate self hosted IRs for each Datafactories been setup, then how do you handle this scenario? As part of your demo even the selfhosted IR node created in Dev will also be deployed to INT & PROD, but what if we have dedicated self hosted IRs to be used for each environment. One thing i observed is the self hosted IR node is not been parameterized as part of ARMTemplateParametersForFactory.json, so how to handle this scenario. I'm actually stuck with this scenario. Could you pls guide me how to handle this?
Thanks much in advance!
Regards,
Nihar
Yes, we have this very case at our company project. This special case, we first deploy the ADF code where we reference the Integration Runtime from the ARM templates. But we go individually and install each individual IR infrastructure in each different environment Data Factories (Dev, Test, Prod).
DeleteSo in this case once the code is deployed to PROD. you manually go and select the prod IR in PROD Data factory?
DeleteYes, that's what plan to do now. It will have to be done once and it doesn't require to change your existing code. Maybe in future this approach may change.
DeleteThanks for your active response!
DeleteHope Microsoft comes up with better solution for this..
I have created the Environment variable inside variable groups as described here and referred to it in the key vault name $(Environment)-kv inside the release pipeline. But it does not recognize $(Environment)! Is there anything more to be set to be able to refer to variables defined inside the variable groups?
ReplyDeleteyou probably have missed below step:
Delete2.i Link Variable groups to Release stages:
Hi Rayis,
ReplyDeleteCould you please help me in getting clarification on below point:
1. If my data factory has multiple pipeline but I checked-in few in Repo, what will happen when I deploy in testing env. Will it deploy all or the checkedin one only?
2. If I made changes to only one component suppose dataset , will ARM deploy the complete factory or the only changed part as part of CI/CD?
Thanks
1) Your GitHub repository (Published branch) will be used a source for deployment. Whatever you manually add to the live data factory shouldn't impact your deployment. However, it's not helping to have your live Data Factory out of sync from your "published" GitHub repo branch.
Delete2) Your development changes will be applied to the publish GitHub branch, although those ARM templates will get recreated. If ARM gets deployed in incremental mode, it does not clean up after itself for objects that have been deleted. This script will help you to clean up: https://docs.microsoft.com/en-us/azure/data-factory/continuous-integration-deployment#sample-script-to-stop-and-restart-triggers-and-clean-up
There is another "Complete" deployment mode for ART templates, but we're not using at our projects, "Incremental" is set by default.
Thank you so much for quick response.
DeleteHello Rayis,
Deletedid you try this MS "script-to-stop-and-restart-triggers-and-clean-up"out?
I am wondering, if I am missing something there, as I could not deploy it in Azure DevOps and I am getting following error:
"The term 'Get-AzDataFactoryV2Trigger' is not recognized as the name of a cmdlet, function, script file, or operable program."
Did you import additional Azure libraries for your PS task in DevOps?
Hello Rayis,
Deletedid you try this MS "script-to-stop-and-restart-triggers-and-clean-up" out?
I am wondering, if I am missing something in my DevOps Task configuration, as I cannot deploy it and I am getting following error:
"The term 'Get-AzDataFactoryV2Trigger' is not recognized as the name of a cmdlet, function, script file, or operable program."
Did you import additional PS libraries in your PS DevOps task?
Hi Eugen, try to use 'Get-AzureRmDataFactoryV2Trigger'. If this doesn't work, try to install the latest version of Azure PowerShell.
DeleteHi Rayis,
ReplyDeleteWhat is your suggestion to implement ADF CI / CD with Azure DevOps using new feature ADF Data Flow. The Agent Azure Data Factory Deployment has no path to "Data Flow"
thanks
Good question, Cezar! Even though that Mapping Data Flows are separate from the rest of pipelines objects in ADF, they can still be added to the deployment ARM Template files. Those Data Flows objects in your ARM template would have the following Type: "type": "Microsoft.DataFactory/factories/dataflows". That means, both Control Flow activities and Mapping Data Flows in ADF can be deployed. I've just checked this in my other Data Factory.
DeleteThank you so much for clear... I'm still working on this configuration in DevOps.
DeleteMy ADF code is in Github enterprise and I can not make a release artifact directly without having a build in step 2.e Add an artifact to your release pipeline.
ReplyDeleteWhile creating a release artifact there are different source types but nothing for Github Enterprise! Any ideas?
Then in this case, create a Build pipeline with a source from your Github enterprise. And then connect this Build pipeline to your release pipeline.
DeleteAt video time 40:26, I am not able to find the service principal of the azure devops to grant access to my Keyvault. Where to get the principal ID ?
ReplyDeleteGood question, I assumed that it got automatically created during my first authentication step between my DevOps project and Azure Subscription at 32:40. And the Principal ID followed the name of my project name itself with some additional GUID value.
DeleteThanks for this great post! It was very helpful.
ReplyDeleteIt seems to me that there is an extra step in ADF releases that the rest of Azure deployments does not have -> manually publishing to the live data factory (which in turn creates the adf_publish branch). Have you thought of automating that step? perhaps a build pipeline that is triggered when the PR is merged into master that runs a script to publish. Which then would trigger the CD pipeline to deploy? Let me know if that doesn't make any sense.
Curious to hear your thoughts!
Thanks again,
Drew
I though about that as well, Drew. And it would be great to have this automation option! If it exists, I'm not aware of. Something that we can ask Microsoft to clarify for us.
DeleteWe have ADFv2 pipelines where we have parameters set to default values. These values need to be changed for test environment and then for production environment.
ReplyDeleteThe parameters list does not show up these parameters, so can you guide on how to proceed with setting up parameters for adfv2 pipeline with values for test and prod
Under Release pipeline, you have Library where you can create Variable Groups with various variables within and then you can assign those Variable groups your different Deployment stages (Dev, Test, UAT, Prod, others). I have this explained in my YouTube video.
DeleteYes those variable groups would be managed for different environments. But it can only be configured for linked service parameters.
DeleteThe pipeline parameters such as source path in your video was a static value for all environments. Suppose, instead of source path is different for instance on test and production environment. How it can be configured in CICD as it does not turn up automatically in template-parameters.json file.
Hope I was able to explain my query here.
https://stackoverflow.com/questions/57894768/how-to-update-adf-pipeline-level-parameters-during-cicd#
ReplyDeleteSame question is been asked on stackoverflow
Those parameters are not exposed in the ARMTemplateParametersForFactory.json file but in the mail ADF file ARMTemplateForFactory.json.
DeleteSeveral ways to make your scenario working:
1) Make ADF pipeline parameter setting part of your configuration:
a) Store different environment parameters in a config file or external DB table
b) Make this config file or DB table part of your DevOps pipeline
2) Find a way to adjust your your ARMTemplateForFactory.json file in CI/CD before deploying to different environments where you set values for your parameters.
Hi Rayis,
ReplyDeleteI am getting below error while implementing Azure adf CI/CD process.
In My data factory I am trying to move the data from on premise to azure sql database using copy task with 2 linked services (one for on premise another for azure Sql ) with self host IR .
So while executing release pipeline I am getting below error. I followed all the steps what you mentioned in above document.
BadRequest: {
"error": {
"code": "BadRequest",
"message": "Failed to encrypt sub-resource payload {\r\n \"Id\": \"/subscriptions/696c1147-0ed1-4fc0-b7dd-36af0533b28f/resourceGroups/ADF/providers/Microsoft.DataFactory/factories/ADFCICDimplementationuat/linkedservices/sourcedb\",\r\n \"Name\": \"sourcedb\",\r\n \"Properties\": {\r\n \"annotations\": [],\r\n \"type\": \"SqlServer\",\r\n \"typeProperties\": {\r\n \"connectionString\": \"********************\",\r\n \"userName\": \"********************\",\r\n \"password\": \"********************\"\r\n },\r\n \"connectVia\": {\r\n \"referenceName\": \"wl-rpt-uat\",\r\n \"type\": \"IntegrationRuntimeReference\"\r\n }\r\n }\r\n} and error is: Invalid linked service payload, please re-input the value for each property..",
"target": "/subscriptions/696c1147-0ed1-4fc0-b7dd-36af0533b28f/resourceGroups/ADF/providers/Microsoft.DataFactory/factories/ADFCICDimplementationuat/linkedservices/sourcedb",
"details": null
}
}
Task failed while creating or updating the template deployment.
Please check if you've enabled Azure DevOps to access your KeyVault.
DeleteThanks for the post, really hopefull.
ReplyDeleteBut just a question, if i have scheduled trigger in my DEV environment and I create this continuous integration system.
Will the trigger be launched in the three environments.
Don't know if i'm clear.
I mean I would like the scheduled trigger to be executed only on the 'Prod' environment, is this possible ?
Hi Rayis.
ReplyDeleteDon't know if my last comment in anonymous was created.
Anyway, first thanks for the work great post.
So know I get a question for you. I want to trigger my pipeline 1 time in a month. So I have created a scheduled trigger. I'm wondering if I am using this continuous integration how does that work ?
Will the scheduled trigger be launched in all the environment "Dev - Test - Prod" (if I have this architecture) because if it's the case that means all three environment will do the same action at the same moment and it's not what I want.
Or is it only the Prod environment which will launch the trigger.
I think quite strange if it's work like this, can you maybe enlighten me ?
Thanks
If you have your trigger enabled at your Development branch, it will also get deployed as enabled, thus could be triggered based on even definition. I would suggest, keep your triggers disabled and then enable them manually in Production after the deployment.
DeleteHi Rayis,
ReplyDeleteI have an issue while I am doing your step.
First I have a resource group where I have my Data Factory resource.
I created my "Service Connection" which is working. But first question, the service manager linked to this service connection should be in the resource group or the data factory resource ?
So I have created my service connection (hopefully the right way), and I chose my Service Connection in the Azure Subscription when I create the Azure Deployment.
Look like I have the right and everything looks fine. But I can't select any "Resource Group", no results found.
Do you know what's wrong ? right issue, wrong service connection ?
Well still I'm trying to put the data factory resource name and when I try to launch the pipeline I get a "Failed to check the resource group status. Error: {"statusCode":403}."
I think the issue come from the fact I can't retrieve any Resource Group when I configure the pipeline.
Do you know where the issue can come from ?
Thaks
Sometimes, it takes a few seconds to refresh a list of available resource groups for your to select. Or you can type the exact name of the resource group, it allows this as well. Please watch my YouTube video on this, I think I've had this case as well.
DeleteI have watched your video nothing linked. And even if I wait, no resource groups arrived. By the way you don't create any Service Connection, I think it must be my issue this Service Connection.
DeleteDid you do any video or test on it ?
Because I can see on your videos that you can have access from 3 Resource Groups for the 3 environment, but I can't understand with Service Connection if you can do the same or how .
If my understanding is correct, before creating release pipeline, we need to have Key vaults for each environment and create two secrets (one for holding storage access key and other for linked service connection string) and we need to place connection string in all three env key vaults before creating release pipeline right?
ReplyDeleteYes, that's correct. Key Vaults with the secrets have to be provisioned first.
DeleteHi Rayis,
ReplyDeleteI have setup the devops pipeline to migrate from dev to prod and it fails with exceed in parameters limit. Most of these parameters are scheduled triggers.
Question :
1. Is there a way I can avoid these scheduled parameters in my
ArmTemplateParameters_master.json ?
2. Or is there any option to proceed with these Triggers.
I see two options: (1) decrease actual number of your triggers, it may require some logic change in your solution as well, (2) with the use of new custom file (arm-template-parameters-definition.json) you can decrease the number of triggers being uploaded into your final ARM template parameter file: https://docs.microsoft.com/en-us/azure/data-factory/continuous-integration-deployment#use-custom-parameters-with-the-resource-manager-template
DeleteHi Rayis, Thank you so much for providing such great articles.
ReplyDeleteI have a question regarding CI CD deployment from Development Azure Data Factory to Production Data factory.
Example: Lets say i have a web activity used in pipelines the web activity URL is connected to logic apps.
In this case how we can customize override parameters for web activities while moving code from Dev to Prod.
Thanks in advance for your response.
Regards,
Ravi.
Hi Ravi, recently Microsoft introduced custom parameters in your ARM deployment templates. And this will help you naturally select all possible JSON elements (including web activities URL) into your ARM template parameter file that you can further override. I've also blogged about this: http://datanrg.blogspot.com/2020/06/raking-custom-parametersvariables-for.html
DeleteThanks for the details. Have you used the process for incremental deployment of ADF with more than 1000 components or using linked templates
ReplyDeleteHi Ashish, I've worked with Linked Templates that had Logic Apps in them, but not with a Data Factory.
DeleteHi,
DeleteWhat if all my ADF’s are git enabled in all environments, hiw this resource manager deployment would behave?
If you connect all ADFs to the same git repository, it will create conflicts when you try to merge code from multiple factories. When you assign each ADF (let's say you have 3-4 data factories for a project) individual git repos, which doesn't make any good sense to me, that you will manage different version of the code in different ADFs separately and you won't need a deployment at all, it will be a mess.
DeleteBest practice: 1 git repo for a project which then you link to your Dev ADF, all the rest of ADF are updated via a automated deployment process using code in your Git Repository.
Hi ,
ReplyDeleteIm facing one issue in ADF deployment with DevOps. Please share your views.
Suppose, i have two Projects pipelines in master. These 2 projects merged into master from respective feature branches. Now i want to promote one project into QA and one into UAT.
How to handle this ? I shud remove first project code then deploy second project into UAT. ?
ADF deployment ARM template and parameter contain full ADF definition. It's a best practice to define one data factory per project and not to mix different project workflows into a single ADF since deployment cycles can be different.
DeleteI have designed the DevOpS release pipeline with DEV ADF(Git configured), QA , UAT and PROD ADF. Each ADF environment will be deployed from adf_publish branch of DEV.
DeleteThis is working fine if we work on one project at a time. But our scenario is multiple developers works in multiple projects by creating feature branch. one projects needs to go live before other. This scenario we are ending up having code that not ready for prod release in adf_publish. How to handle this? Our projects are not so big to create separate ADF. I also tried Revert PR option, but after Reverting not able to merge the same feature branch back to master in DEV.
Hi Swapna, I understand your concern. Don't look at a single Data Factory as a big resource that should combine all ETL processes of all the projects (small and big) that you can think of. I believe that ADF is similar to an SSIS projects, and all components in one ADF should share the same deployment cycle. Plus from the cost perspective it will be better to segregate workload for each project separately, i.e. having multiple projects in a single ADF is not a best practice.
DeleteIt is a great blog engaging DevOps practices. I would like to add one more things that DevOps is a set of software development practices that combines software development (Dev) and information technology operations (Ops) to optimise the delivery of a product, solution or platform.
ReplyDeleteonce the code repo is synced , is there any way to deploy the updated codes to the respective datafactory resources without any conflicts and deletion of artifacts that is not present in code repo but present in adf.
ReplyDeleteThese are still unsupported CICD features of ADF: https://docs.microsoft.com/en-us/azure/data-factory/continuous-integration-delivery#unsupported-features
DeleteYou can read about more pre and post deployment script to avoid any potential conflicts during the deployment: https://docs.microsoft.com/en-us/azure/data-factory/continuous-integration-delivery-sample-script
DeleteHow do you stop the deploy flow from Dev to QA to PRD, when the linkedsevices type sftp uses different hosts? In my scenario when I deploy DEV to QA to PROD the host that exists in QA and PROD are changed by the host that is in DEV, is there a way to block this?
ReplyDeleteHow do you stop the deploy flow from Dev to QA to PRD, when the linkedsevices type sftp uses different hosts? In my scenario when I deploy DEV to QA to PROD the host that exists in QA and PROD are changed by the host that is in DEV, is there a way to block this?
ReplyDeleteThis is simple, you will just need to override required parameters from the ArmTemplate Parameter file and replace their initial DEV values for the QA and PROD stages during the deployment. I explain that in the video and my blog post (2.f Add new Stage to your Release pipeline).
Delete